Batch Processing of tumor biopsies for cell markers
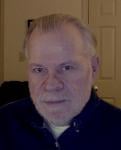
Randomized International Phase III Trial of ERCC1 and RRM1 Expression–Based Chemotherapy Versus Gemcitabine/Carboplatin in Advanced Non–Small-Cell Lung Cancer
Gerold Bepler, Charles Williams, Michael J. Schell, Wei Chen, Zhong Zheng, George Simon, Shirish Gadgeel, Xiuhua Zhao, Fred Schreiber, Julie Brahmer, Alberto Chiappori, Tawee Tanvetyanon, Mary Pinder-Schenck, Jhanelle Gray, Eric Haura, Scott Antonia, and Juergen R. Fischer
Abstract
Purpose:
We assessed whether chemotherapy selection based on in situ ERCC1 and RRM1 protein levels would improve survival in patients with advanced non–small-cell lung cancer (NSCLC).
Patients and Methods:
Eligible patients were randomly assigned 2:1 to the trial’s experimental arm, which consisted of gemcitabine/carboplatin if RRM1 and ERCC1 were low, docetaxel/carboplatin if RRM1 was high and ERCC1 was low, gemcitabine/docetaxel if RRM1 was low and ERCC1 was high, and docetaxel/vinorelbine if both were high. In the control arm, patients received gemcitabine/ carboplatin. The trial was powered for a 32% improvement in 6-month progression-free sur- vival (PFS).
Results:
Of 331 patients registered, 275 were eligible. The median number of cycles given was four in both arms. A tumor rebiopsy specifically for expression analysis was required in 17% of patients. The median time from informed consent to expression analysis was 11 days. We found no statistically significant differences between the experimental arm and the control arm in PFS (6.1 months v 6.9 months) or overall survival (11.0 months v 11.3 months). A subset analysis revealed that patients with low levels for both proteins who received the same treatment in both treatment arms had a statistically better PFS (P = .02) in the control arm (8.1 months) compared with the experimental arm (5.0 months).
Conclusion:
This demonstrates that protein expression analysis for therapeutic decision making is feasible in newly diagnosed patients with advanced-stage NSCLC. A tumor rebiopsy is safe, required in 17%, and acceptable to 89% (47 of 53) of patients.
J Clin Oncol 31:2404-2412. 2013 by American Society of Clinical Oncology
http://www.ncbi.nlm.nih.gov/pubmed/23690416
Comments
-
Fatal Flaw
In another prospective study, genomic testing was prognostic for response to anthracycline and taxane therapy in women with newly diagnosed invasive breast cancer was shown to have improved on predictions based on clinicopathologic parameters.
Here's the fatal flaw: It's just like all similar studies (including OncotypeDX). It's not a real world situation. What they are doing is to collect and freeze specimens and batch process them. This is similar to virtually all of the breast cancer studies, using IHC for ER and Her2 (where studies are performed by batch processing archival, paraffin-embedded specimens).
This is not real world, in which specimens are received daily, in real time, and processed in a clinically relevant time frame. In the real world, when patient gets a biopsy, the specimen gets processed and reported within a few days to a couple of weeks. Each specimen is processed and tested individually, by whomever happens to be working on the days in question. It's not the same team of technicians, working with the same pathologist, with the same reagents and the same microarrays, doing it all at the same time.
With cell culture assays, it is a real world situation. Specimens are received over days, weeks, months, years. They are processed and completed as they come in through the door.
With the NEJM OncotypeDX study, all of the specimens were batched processed within the same 2 week time segment (for hundreds of specimens). Had the specimens been processed and studied under real world conditions (months and years), the correlations would certainly not have been nearly as good.
The same thing goes with ER and Her2. These are "batch processed" studies. This is the only study of which there was reasonably real world (specimens processed and tests completed as specimens were received). Note the poor correlations, particularly with regard to false negatives.
I don't know how one could really deny any breast cancer patient with metastatic disease a trial of hormonal therapy, given the 20% response rate for ER negative patients, with ER performed using IHC in "real world" conditions. But we think that ER negative patients have only a 10% or less response rate, based entirely on non-real world "batch processed" studies.
But that's how all these multi-gene studies are done. Batch processed and retrospective. Utterly non-real world.
Private laboratory oncologists have been making this point for years...finally validated -- in the JCO, no less!
All of these marker studies (including Her2, ER, KRAS, EGFR mutations, OncotypeDx, etc.) are highly artificial, non-real world studies. Everything gets batch processed by the same crack team of technologists, same reagents, same platforms, same pathologists, etc. over a brief period of time. In cell culture studies, they are constrained to "real world conditions." Specimens processed and tested as accessioned, in real time, over days, weeks, months, years.
In this study, the authors got null results, which didn't agree with previous findings, and blamed this on the fact that the present study was "real world," while all the prior studies were "batch processed."
From their discussion:
"Finally, it is important to note, that our study required real- time processing of tumor specimens for ERCC1 and RRM1 in situ protein levels. All prior investigations of these molecules utilized batch processing of tumor samples. Thus, day-to-day variations in the assay reliability may have not affected prior investigations, whereas our investigation suffered from this. During the entire trial, all specimens were processed by one of two investigators using a standardized operating procedure, device, and image anal- ysis application. Reagents were from similar sources and prepared identically; however, different lots of reagents were used during the 3.5-year patient accrual period. In an analysis of ERCC1 and RRM1 values over time, we noticed nonrandom trends in marker levels, suggesting that reagent and processing procedures may have influ- enced the biomarker levels.
In summary, we believe that the survival results and possibly the disease response results are false negative. However, the trial clearly demonstrates feasibility of treatment assignment for patients with advanced NSCLC across countries and academic, nonacademic, and private practice settings. We conclude that further assay development with special attention to reagent specificity, day-to-day assay conditions, and site-specific specimen processing is desirable before another trial is launched."0 -
Type One Errorgdpawel said:Fatal Flaw
In another prospective study, genomic testing was prognostic for response to anthracycline and taxane therapy in women with newly diagnosed invasive breast cancer was shown to have improved on predictions based on clinicopathologic parameters.
Here's the fatal flaw: It's just like all similar studies (including OncotypeDX). It's not a real world situation. What they are doing is to collect and freeze specimens and batch process them. This is similar to virtually all of the breast cancer studies, using IHC for ER and Her2 (where studies are performed by batch processing archival, paraffin-embedded specimens).
This is not real world, in which specimens are received daily, in real time, and processed in a clinically relevant time frame. In the real world, when patient gets a biopsy, the specimen gets processed and reported within a few days to a couple of weeks. Each specimen is processed and tested individually, by whomever happens to be working on the days in question. It's not the same team of technicians, working with the same pathologist, with the same reagents and the same microarrays, doing it all at the same time.
With cell culture assays, it is a real world situation. Specimens are received over days, weeks, months, years. They are processed and completed as they come in through the door.
With the NEJM OncotypeDX study, all of the specimens were batched processed within the same 2 week time segment (for hundreds of specimens). Had the specimens been processed and studied under real world conditions (months and years), the correlations would certainly not have been nearly as good.
The same thing goes with ER and Her2. These are "batch processed" studies. This is the only study of which there was reasonably real world (specimens processed and tests completed as specimens were received). Note the poor correlations, particularly with regard to false negatives.
I don't know how one could really deny any breast cancer patient with metastatic disease a trial of hormonal therapy, given the 20% response rate for ER negative patients, with ER performed using IHC in "real world" conditions. But we think that ER negative patients have only a 10% or less response rate, based entirely on non-real world "batch processed" studies.
But that's how all these multi-gene studies are done. Batch processed and retrospective. Utterly non-real world.
Private laboratory oncologists have been making this point for years...finally validated -- in the JCO, no less!
All of these marker studies (including Her2, ER, KRAS, EGFR mutations, OncotypeDx, etc.) are highly artificial, non-real world studies. Everything gets batch processed by the same crack team of technologists, same reagents, same platforms, same pathologists, etc. over a brief period of time. In cell culture studies, they are constrained to "real world conditions." Specimens processed and tested as accessioned, in real time, over days, weeks, months, years.
In this study, the authors got null results, which didn't agree with previous findings, and blamed this on the fact that the present study was "real world," while all the prior studies were "batch processed."
From their discussion:
"Finally, it is important to note, that our study required real- time processing of tumor specimens for ERCC1 and RRM1 in situ protein levels. All prior investigations of these molecules utilized batch processing of tumor samples. Thus, day-to-day variations in the assay reliability may have not affected prior investigations, whereas our investigation suffered from this. During the entire trial, all specimens were processed by one of two investigators using a standardized operating procedure, device, and image anal- ysis application. Reagents were from similar sources and prepared identically; however, different lots of reagents were used during the 3.5-year patient accrual period. In an analysis of ERCC1 and RRM1 values over time, we noticed nonrandom trends in marker levels, suggesting that reagent and processing procedures may have influ- enced the biomarker levels.
In summary, we believe that the survival results and possibly the disease response results are false negative. However, the trial clearly demonstrates feasibility of treatment assignment for patients with advanced NSCLC across countries and academic, nonacademic, and private practice settings. We conclude that further assay development with special attention to reagent specificity, day-to-day assay conditions, and site-specific specimen processing is desirable before another trial is launched."Hypothesis testing is based on certain statistical and mathematical principles that allow investigators to evaluate data by making decisions based on the probability or implausibility of observing the results obtained.
However, classic hypothesis testing has its limitations, and probabilities mathematically calculated are inextricably linked to sample size.
Furthermore, the meaning of the p value frequently is misconstrued as indicating that the findings are also of clinical significance.
Finally, hypothesis testing allows for four possible outcomes, two of which are errors that can lead to erroneous adoption of certain hypotheses:
1. The null hypothesis is rejected when, in fact, it is false.
2. The null hypothesis is rejected when, in fact, it is true (type I or alpha error).
3. The null hypothesis is conceded when, in fact, it is true.
4. The null hypothesis is conceded when, in fact, it is false (type II or beta error).
Type I error occurs when you CANNOT reject the null hypothesis and type II error occurs when you reject it inappropriately. The other two outcome would be consistent with what you might look upon as true positives and true negatives.
The sample size error is extremely important for it goes to the next point of all these discussions
That is
When does statistical significance occur and not be relevant and when does statistical significance not occur and yet the actual finding prove to be of great relevance. Sample size dictates that.0 -
Run Batch Effects Compromise Usefulness of Genomic Signaturesgdpawel said:Type One Error
Hypothesis testing is based on certain statistical and mathematical principles that allow investigators to evaluate data by making decisions based on the probability or implausibility of observing the results obtained.
However, classic hypothesis testing has its limitations, and probabilities mathematically calculated are inextricably linked to sample size.
Furthermore, the meaning of the p value frequently is misconstrued as indicating that the findings are also of clinical significance.
Finally, hypothesis testing allows for four possible outcomes, two of which are errors that can lead to erroneous adoption of certain hypotheses:
1. The null hypothesis is rejected when, in fact, it is false.
2. The null hypothesis is rejected when, in fact, it is true (type I or alpha error).
3. The null hypothesis is conceded when, in fact, it is true.
4. The null hypothesis is conceded when, in fact, it is false (type II or beta error).
Type I error occurs when you CANNOT reject the null hypothesis and type II error occurs when you reject it inappropriately. The other two outcome would be consistent with what you might look upon as true positives and true negatives.
The sample size error is extremely important for it goes to the next point of all these discussions
That is
When does statistical significance occur and not be relevant and when does statistical significance not occur and yet the actual finding prove to be of great relevance. Sample size dictates that.Run Batch Effects Potentially Compromise the Usefulness of Genomic Signatures for Ovarian Cancer
Keith A. Baggerly, Kevin R. Coombes, and E. Shannon Neeley
Department of Bioinformatics & Computational Biology, University of Texas M.D. Anderson Cancer Center, Houston, TX
JCO March 1, 2008:1186-1187; DOI:10.1200/JCO.2007.15.1951.
Editor:
A major goal of personalized medicine is to predict, before administering a treatment, whether the patient will respond to it. Recently, Dressman et al1 presented an approach that appeared to move us toward this goal in the context of ovarian cancer. Using microarray expression profiles, they first identified a set of genes that could differentiate between patients who did (CR) and did not (NR) respond to primary platinum-based chemotherapy. Then, following Bild et al,2 they scored each tumor for the levels of five different oncogenic pathways. They reported that three pathways (Src, E2F3, and Myc) stratify the NRs into subgroups with significantly different survival characteristics, suggesting how further therapies might be targeted for these patients.
We examined these data in order to help investigators at our institution make better use of this approach. We were unable to reproduce the results reported, and the structure that we did find appears driven far more by run date than by clinical response. Our findings are outlined here; supplementary reports (ovca01-ovca07) provide details.
(1) The posted mapping of numbers to samples is scrambled (eg, numbers from sample 872 are labeled as belonging to sample 2476). Only 32 of the 119 mappings are correct. Three quantifications were derived from raw array data (CEL files) not used for this study (ovca01). Whether this scrambling is fatal depends on when it occurred, which we cannot assess.
(2) We assembled clinical data by combining information on subsets of the samples from Dressman et al,1 Bild et al,2 and Berchuck et al.3 Survival status changes for 15 patients in going from Bild et al2 to Dressman et al1 revealed that 14 CR patients shifted from Alive to Dead, and one NR patient shifted from Dead to Alive. Information from Berchuck et al3 suggests that the Bild et al2 annotation is correct (ovca02).
(3) We identified 107 Affymetrix (Santa Clara, CA) probeset IDs corresponding to the “best” 100 genes reported by Dressman et al1; ambiguities in annotation led to some duplication (ovca03).
(4) The CEL files can be grouped into clearly separated batches on the basis of run date. Response and survival are confounded with run date, particularly with the samples processed earliest (ovca04).
(5) We contrasted the CR and NR samples, gene by gene, using two-sample t tests. P values from the reported “best 100” genes are uniformly distributed, suggesting results no better than chance. Clustering based on these genes fails to separate CRs from NRs. There is some evidence of differential expression in the set of all genes. Gene-by-gene analyses of variance, however, suggest strong batch effects for almost every gene. After correcting for these batch effects, separation between CRs and NRs drops to low levels (ovca05).
(6) Using data from Bild et al,2 we computed our own pathway scores for each tumor sample. Our pathway gene lists differ slightly from those of Bild et al2 due to differences in array processing (Affymetrix Microarray Analysis Suite, v.5.0 in Bild et al,2 robust multi-array analysis here). These scores are relatively robust with respect to the precise gene list selected, but they show clear confounding with run batch. After correcting for batch, the scores change substantially (ovca06).
(7) Finally, we looked for differences in survival as a function of dichotomized (high/low) pathway scores. For each pathway, we looked at results for three patient subgroups (NR, CR, and all) using all combinations of (a) our quantifications or those reported, (b) our gene list or those reported, (c) ignoring or correcting for run batch, and (d) censoring according to Dressman et al1 or Bild et al.2 After correcting for batch, the only contrasts that remain modestly significant involve E2F3 and the patient subgroups of CRs or all, though the P values do not take multiple testing into account (ovca07).
Batch effects are common in large-scale expression studies, but are not commonly addressed. When such batches are confounded with biologic contrasts of interest, problems can arise. Fortunately, as noted in Ransohoff,4 these problems can be somewhat circumvented through good experimental design. Further, batch effects can be modeled if we remember to look for them.
We would be delighted if the methods and results outlined in Dressman et al1 worked. Unfortunately, based on the results shown in this analysis, we are not yet persuaded that either the signature or the pathway stratification will lead to better patient care. While there may be differences due to pathway activation, run batch effects provide an alternative explanation here, and in our experience, batch effects are often larger than biologic ones.
Details of our analysis, including our code, documentation, figures, and results are available from http://bioinformatics.mdanderson.org/Supplements/ReproRsch-Ovary/
Given the software (freeware statistical package R version 2.5.1) and our code, all the results we report are reproducible.
References:
1. Dressman HK, Berchuck A, Chan G, et al: An integrated genomic-based approach to individualized treatment of patients with advanced-stage ovarian cancer. J Clin Oncol 25:517-525, 2007
2. Bild A, Yao G, Chang JT, et al: Oncogenic pathway signatures in human cancers as a guide to targeted therapies. Nature 439:353-357, 2006
3. Berchuck A, Iversen ES, Lancaster JM, et al: Patterns of gene expression that characterize long term survival in advanced serous ovarian cancers. Clin Cancer Res 11:3686-3696, 2005
4. Ransohoff DF: Bias as a threat to the validity of cancer molecular-marker research. Nat Rev Cancer 5:142-149, 2005
Note: In this case, the problem is much more serious. This was the Duke University group (Potti the lead, dishonest, fraudulent investigator), who's work was later exposed as being grossly fraudulent and this paper, as well as many others from this group, was formally retracted.
http://jco.ascopubs.org/content/30/6/678
The results of a particular clinical trial may be true only for the batch that is used in the study. For example, batch effects, introduced by profiling samples on different days using different lots of reagents or at different sites, can introduce variations and confound such analyses. These considerations require reproducing the classification results in independent test cohorts of samples and using multiple hypothesis testing correction methods. In other words, if batch effects are not controlled, it may lead to spurious findings (Genes & Dev. 2011.25:534-555).0 -
Thank you.gdpawel said:Run Batch Effects Compromise Usefulness of Genomic Signatures
Run Batch Effects Potentially Compromise the Usefulness of Genomic Signatures for Ovarian Cancer
Keith A. Baggerly, Kevin R. Coombes, and E. Shannon Neeley
Department of Bioinformatics & Computational Biology, University of Texas M.D. Anderson Cancer Center, Houston, TX
JCO March 1, 2008:1186-1187; DOI:10.1200/JCO.2007.15.1951.
Editor:
A major goal of personalized medicine is to predict, before administering a treatment, whether the patient will respond to it. Recently, Dressman et al1 presented an approach that appeared to move us toward this goal in the context of ovarian cancer. Using microarray expression profiles, they first identified a set of genes that could differentiate between patients who did (CR) and did not (NR) respond to primary platinum-based chemotherapy. Then, following Bild et al,2 they scored each tumor for the levels of five different oncogenic pathways. They reported that three pathways (Src, E2F3, and Myc) stratify the NRs into subgroups with significantly different survival characteristics, suggesting how further therapies might be targeted for these patients.
We examined these data in order to help investigators at our institution make better use of this approach. We were unable to reproduce the results reported, and the structure that we did find appears driven far more by run date than by clinical response. Our findings are outlined here; supplementary reports (ovca01-ovca07) provide details.
(1) The posted mapping of numbers to samples is scrambled (eg, numbers from sample 872 are labeled as belonging to sample 2476). Only 32 of the 119 mappings are correct. Three quantifications were derived from raw array data (CEL files) not used for this study (ovca01). Whether this scrambling is fatal depends on when it occurred, which we cannot assess.
(2) We assembled clinical data by combining information on subsets of the samples from Dressman et al,1 Bild et al,2 and Berchuck et al.3 Survival status changes for 15 patients in going from Bild et al2 to Dressman et al1 revealed that 14 CR patients shifted from Alive to Dead, and one NR patient shifted from Dead to Alive. Information from Berchuck et al3 suggests that the Bild et al2 annotation is correct (ovca02).
(3) We identified 107 Affymetrix (Santa Clara, CA) probeset IDs corresponding to the “best” 100 genes reported by Dressman et al1; ambiguities in annotation led to some duplication (ovca03).
(4) The CEL files can be grouped into clearly separated batches on the basis of run date. Response and survival are confounded with run date, particularly with the samples processed earliest (ovca04).
(5) We contrasted the CR and NR samples, gene by gene, using two-sample t tests. P values from the reported “best 100” genes are uniformly distributed, suggesting results no better than chance. Clustering based on these genes fails to separate CRs from NRs. There is some evidence of differential expression in the set of all genes. Gene-by-gene analyses of variance, however, suggest strong batch effects for almost every gene. After correcting for these batch effects, separation between CRs and NRs drops to low levels (ovca05).
(6) Using data from Bild et al,2 we computed our own pathway scores for each tumor sample. Our pathway gene lists differ slightly from those of Bild et al2 due to differences in array processing (Affymetrix Microarray Analysis Suite, v.5.0 in Bild et al,2 robust multi-array analysis here). These scores are relatively robust with respect to the precise gene list selected, but they show clear confounding with run batch. After correcting for batch, the scores change substantially (ovca06).
(7) Finally, we looked for differences in survival as a function of dichotomized (high/low) pathway scores. For each pathway, we looked at results for three patient subgroups (NR, CR, and all) using all combinations of (a) our quantifications or those reported, (b) our gene list or those reported, (c) ignoring or correcting for run batch, and (d) censoring according to Dressman et al1 or Bild et al.2 After correcting for batch, the only contrasts that remain modestly significant involve E2F3 and the patient subgroups of CRs or all, though the P values do not take multiple testing into account (ovca07).
Batch effects are common in large-scale expression studies, but are not commonly addressed. When such batches are confounded with biologic contrasts of interest, problems can arise. Fortunately, as noted in Ransohoff,4 these problems can be somewhat circumvented through good experimental design. Further, batch effects can be modeled if we remember to look for them.
We would be delighted if the methods and results outlined in Dressman et al1 worked. Unfortunately, based on the results shown in this analysis, we are not yet persuaded that either the signature or the pathway stratification will lead to better patient care. While there may be differences due to pathway activation, run batch effects provide an alternative explanation here, and in our experience, batch effects are often larger than biologic ones.
Details of our analysis, including our code, documentation, figures, and results are available from http://bioinformatics.mdanderson.org/Supplements/ReproRsch-Ovary/
Given the software (freeware statistical package R version 2.5.1) and our code, all the results we report are reproducible.
References:
1. Dressman HK, Berchuck A, Chan G, et al: An integrated genomic-based approach to individualized treatment of patients with advanced-stage ovarian cancer. J Clin Oncol 25:517-525, 2007
2. Bild A, Yao G, Chang JT, et al: Oncogenic pathway signatures in human cancers as a guide to targeted therapies. Nature 439:353-357, 2006
3. Berchuck A, Iversen ES, Lancaster JM, et al: Patterns of gene expression that characterize long term survival in advanced serous ovarian cancers. Clin Cancer Res 11:3686-3696, 2005
4. Ransohoff DF: Bias as a threat to the validity of cancer molecular-marker research. Nat Rev Cancer 5:142-149, 2005
Note: In this case, the problem is much more serious. This was the Duke University group (Potti the lead, dishonest, fraudulent investigator), who's work was later exposed as being grossly fraudulent and this paper, as well as many others from this group, was formally retracted.
http://jco.ascopubs.org/content/30/6/678
The results of a particular clinical trial may be true only for the batch that is used in the study. For example, batch effects, introduced by profiling samples on different days using different lots of reagents or at different sites, can introduce variations and confound such analyses. These considerations require reproducing the classification results in independent test cohorts of samples and using multiple hypothesis testing correction methods. In other words, if batch effects are not controlled, it may lead to spurious findings (Genes & Dev. 2011.25:534-555).It's good to know we can count on you to provide us with the most up to date information.
0
Discussion Boards
- All Discussion Boards
- 6 CSN Information
- 6 Welcome to CSN
- 121.2K Cancer specific
- 2.8K Anal Cancer
- 440 Bladder Cancer
- 306 Bone Cancers
- 1.6K Brain Cancer
- 28.4K Breast Cancer
- 391 Childhood Cancers
- 27.9K Colorectal Cancer
- 4.6K Esophageal Cancer
- 1.2K Gynecological Cancers (other than ovarian and uterine)
- 12.9K Head and Neck Cancer
- 6.3K Kidney Cancer
- 666 Leukemia
- 789 Liver Cancer
- 4.1K Lung Cancer
- 5.1K Lymphoma (Hodgkin and Non-Hodgkin)
- 235 Multiple Myeloma
- 7.1K Ovarian Cancer
- 53 Pancreatic Cancer
- 486 Peritoneal Cancer
- 5.3K Prostate Cancer
- 1.2K Rare and Other Cancers
- 532 Sarcoma
- 717 Skin Cancer
- 647 Stomach Cancer
- 190 Testicular Cancer
- 1.5K Thyroid Cancer
- 5.8K Uterine/Endometrial Cancer
- 6.3K Lifestyle Discussion Boards